Top 10 Things Every Senior Decision-Maker Should Know About AI
From the Big Picture to the Personal Toolbox—A Guide to Corporate Applications of Generative Artificial Intelligence
Over the past two years, AI has evolved from a limited corporate curiosity, to a central driver of business strategy. Conversations have moved beyond step one: explaining the underlying technologies, speculating about existential risks, and mocking chatbots’ limitations. Then, it was led by visionary volunteers. Now, companies are taking the lead, with the objective of integrating AI across the organizations. However, despite the shift, most companies have yet to realize substantial, enterprise-wide impact from AI.
Exceptions to the slow pace of AI adoption is found in marketing, analytics, and software development. Today, nearly all programmers leverage AI to enhance their productivity. However, similar effects have yet to extend across other business functions. Interestingly, AI’s early impact has been most significant for less experienced workers, helping them close the gap with top performers, who have seen limited gains, if any.
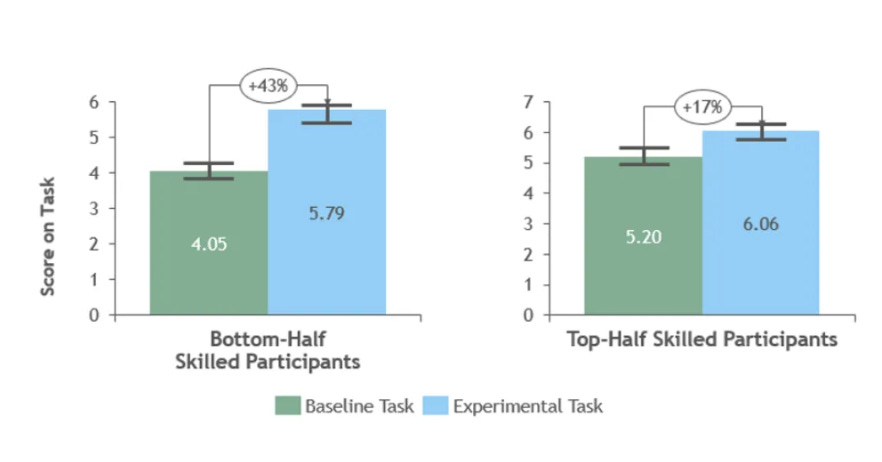
As of fall 2024, the AI landscape is poised for significant change. As frontier models, projected to be 100 times more powerful than the original GPT-4 approach, the competitive landscape may shift once again. Whether these advances will drive widespread improvements across industries or just act as a performance equalizer remains uncertain.
Given these dynamics, what should CEOs prioritize, as AI’s role in business continues to evolve? To help answer that question, I have compiled the top 10 things every senior decision-maker should know about AI today.
Part I: Understanding the Trends and Potential
While your new smartphone may only be marginally better than last year’s model, today’s large language models (LLMs) have improved tenfold—a trend that shows no immediate signs of slowing. This rapid advancement has persisted for some years, fuelled by both technological breakthroughs and substantial financial investments. Factors like anticipated energy constraints may, however, slow AI progress by decade’s end, while others factors, such as AI’s role in its own development, could accelerate it. Though tenfold annual growth won’t continue indefinitely, three more years at this rate would produce a thousandfold improvement. History shows that exponential trends—like those observed in Moore’s Law, that has accurately predicted microchip development for 50 years—can persist for a long time, before inevitably levelling off.
#1. The AI Bubble: Is AI a Bubble Ready to Pop?
Media and analysts increasingly reference an 'AI bubble'. Typically, this is referring to AI market valuation bubble. More specific, they are talking about Nvidia, NVDA, the leading GPU provider and the world’s second-largest company by market capitalization. With Nvidia's rapid growth and inherent market uncertainties, significant stock price volatility should be anticipated. While a price drop may prompt claims of an AI bubble bursting, extending this to imply that AI technology is a bubble is a stretch.
Yet, claims of AI’s technological demise persist, often voiced by economists like Goldman Sachs’s Jim Covello or MIT Nobel laureate Daron Acemoğlu. It’s important to note that their projections aren’t based on the technological roadmaps from AI lab insiders. Instead, they rely on their own technical assumptions, presuming that AI progress will abruptly halt. In my view, Acemoğlu has largely misjudged AI development timelines, while Covello lacks a deeper technology understanding, even suggesting that the shift from cell phones to smartphones was a greater leap than the invention of artificial intelligence itself.
Just seven weeks after the Goldman Sachs report, OpenAI released its o1 models, capable of graduate-level reasoning—a milestone neither Acemoğlu nor Covello anticipated within the next decade, if ever. So, while these kind of analyses will have a short shelf life, high media demand for pessimistic AI projections ensures their persistence.
The pessimistic view common among economists, journalists, and other non-AI-experts isn’t entirely unfounded. An often referred to hypothesis suggests that LLMs face inherent technological limitations that could rapidly diminish returns as models scale. This perspective is championed by a small but vocal group, notably Yann LeCun at Meta and François Chollet at Google, who argue that LLMs can only generalize narrowly. They claim that the abilities of LLMs to build models of the world, and their apparent emergent properties, are illusions. They claim that the technology is essentially performing advanced pattern matching and extrapolation. According to this view, LLMs represent a technological dead end, and future models in the ‘GPT-5 era’ will be only marginally better than those today. Most insiders in AI labs disagree, as data so far does not generally support this hypothesis. However, the upcoming release of GPT-5 may provide more clarity.
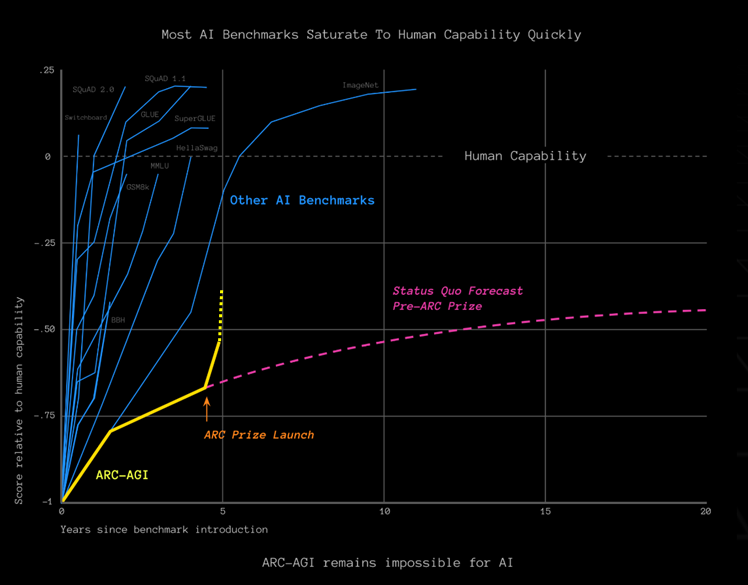
In the European Union—and especially in Sweden, where I live—mainstream media typically portrays digitalization and AI with a negative bias. Microsoft has identified a ‘fear of digitalization’ in Sweden, leading to lower public trust, reduced AI adoption, and ultimately a decline in national competitiveness. As a consequence, companies tend to avoid any risks associated with using generative AI. However, at the same time they are exposing themselves to the much larger risks of being left behind. The real ‘bubble’ to watch may be the information bubble of pervasive negativity, rather than an AI technology bubble.
#2. The AI Nothingburger: Why Has Generative AI's Business Impact Been Less Than Expected?
Recent data shows that a quarter of adults in the U.S. used generative AI in their work over the past week, and corporate adoption is accelerating far faster than with previous technologies, such as computers or the internet. Yet, on a macro scale, AI adoption has not yet translated into substantial productivity gains or GDP growth.
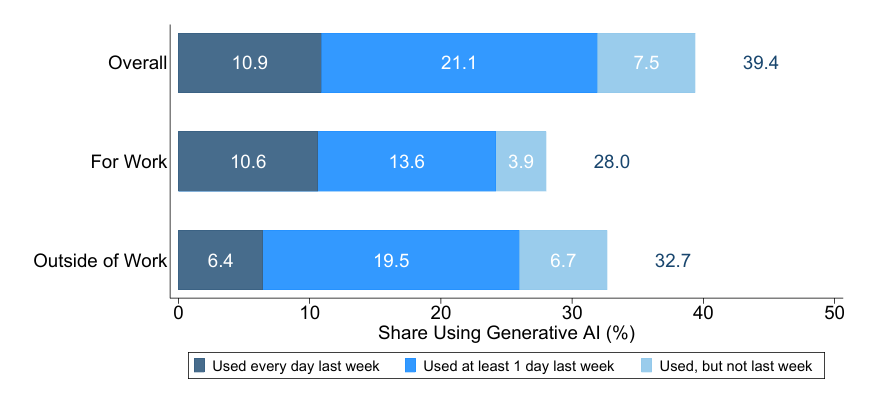
One factor limiting AI’s impact is that companies have yet to fully leverage this technology. Organizational inertia hinders change, and implementing a new technology that affects every process and capability is particularly challenging. Furthermore, a lack of training and understanding among leaders and employees on how to use AI effectively limits adoption. This is compounded by concerns around misuse, data security, regulatory compliance, and ethics.
Many companies have also yet to feel competitive pressure to act, invest, and take risks with AI. Although some may be lagging behind peers in AI adoption, this has not yet negatively impacted their cost structures, triggered customer loss, or caused employees to leave. However, this may only be a matter of time.
A technical factor also plays a role: until recently, AI tools lacked the capabilities needed to deliver significant value. I previously discussed this in “The Failed Promise of Corporate AI Productivity,” highlighting the challenges Microsoft faced in integrating intelligence into its Office suite. It wasn’t until the release of Microsoft 365 Copilot Wave 2 on September 16, 2024, that we began to see practical, value-adding use cases emerge.
The limited impact of generative AI on business is likely temporary. But, understanding this requires thinking in terms of exponential growth. With underlying AI technology advancing at a rate of 10x per year, progress effectively doubles roughly every 3.5 months. This means that in the next 3.5 months, we can expect as much development as we’ve seen cumulatively since the invention of generative AI.
#3. Human-Level AI Approaching: What Does AGI Mean for Your Business, and When Will It Arrive?
The pivotal threshold will be when AI reaches human-level performance, making it interchangeable with humans across various job roles. This is encapsulated in the controversial term “AGI,” or Artificial General Intelligence.
The bar for AGI keeps rising. Just a few years ago, AGI was defined as the ability to answer questions at the level of an average human. Today, it implies the capacity to perform any conceivable task a remote worker could handle, and to do so as well as any human. Even by this high standard, most insiders expect AGI to be achieved by 2027, or at least within the next five years.
It’s worth noting that for OpenAI, ‘AGI’ is also a contractual milestone with its investors. Specifically, Microsoft’s rights to OpenAI’s models extend only to the point at which AGI is achieved. The decision on when AGI is achieved lies with OpenAI’s Board. Consequently, Microsoft has an especially high bar for what they consider qualifies as AGI:
“AGI may even take us beyond our planet by unlocking the doors to space exploration; it could help us develop interstellar technology and identify and terraform potentially habitable exoplanets. It might even shed light on the origins of life and the universe.”
Most would classify this as ASI, or Artificial Superintelligence, which surpasses the collective human intelligence. OpenAI, however, uses its own five-level definition for AGI. Following the release of the o1 model, OpenAI believes progress has advanced from level 1 to level 2 and anticipates reaching level 3 shortly. At level 5, AI would be capable of autonomously running companies and organizations without human intervention.
Although ‘AGI’ is a useful term for setting expectations, I don’t foresee a single ‘AGI moment’ when everyone universally acknowledges its arrival. Instead, I believe AGI will be something we discover in the rear-view mirror.
Part II: The Corporate Big Picture
Few companies fully grasp the potential impact of approaching AGI on their business. It’s important to understand that AGI isn’t about chatbots; it’s expected to function as a network of intelligent agents. Rather than interacting by asking questions, users will assign these agents specific goals. Initially, agents might handle tasks like “plan a weekend in Paris, book all necessary arrangements, and tailor the itinerary to my preferences.” However, the complexity of tasks AGI agents can accomplish is expected to escalate rapidly. Companies will need to treat these agents more like employees—granting them email addresses, data access, and roles within the organization—though with the advantage of operating 24/7 and handling certain tasks at superhuman speeds.
#4. The Wait-and-See Approach: Can You Afford to Slow Roll AI?
With level 5 AI agents on the horizon—potentially capable of autonomously running entire companies—it’s fair to wonder: Why invest in building AI capabilities and training employees now? Why not just wait for AGI to fully mature? While a valid question, a ‘slow-roll’ strategy is likely not ideal.
First, AI progress may not unfold exactly in a way where agents can suddenly take over all tasks. Another probable scenario involves a phased transition, where hybrid models emerge, with certain roles remaining human-led for the foreseeable future. To prepare, companies need a robust, adaptable strategy—not one based on a single, specific outcome. This requires the skilled personnel, strategic hires, and integration plans, as well as a gradual transition beginning now.
The second reason to avoid a ‘slow-roll’ approach is AI’s dual-use potential. Cybersecurity is a prime example where AI capabilities are critical. Delaying AI adoption can leave companies vulnerable to increasingly sophisticated threats. For instance, competitors could deploy thousands of AI agents to discredit your brand across social media or execute advanced social engineering attacks targeting key employees to disrupt or steal trade secrets. Effectively countering these risks requires AI-driven defenses embedded as a company-wide capability.
So, in practice, any "wait-and-see" approach is a high-risk strategy, likely to fail. Speed and agility will be critical success factors. With AI evolving rapidly, each day of delay makes catching up more difficult. And even if your organization has started integrating AI, staying competitive won’t be easy. Global competition won’t be evenly matched; some companies and countries will inevitably pull ahead. Additionally, access to AI models may not be universal—certain models could be restricted outside the U.S., for instance—forcing companies in regions like the EU to rely on entities in the U.S. or alternative sources.
#5. Beyond Programmers and Chatbots: What's the Next Step in Corporate AI?
Today’s most effective corporate AI use cases include software development, customer care, service, and support functions, such as chatbots.
The next step requires AI agents that can operate more autonomously, similar to human employees. AI agents are emerging, especially in software development. However, more advanced foundational models are needed to prevent error accumulation in these agents.
In the near future, expect to hear more about ‘AI agent swarms.’ While it may sound complex, agent swarms are essentially teams of specialized AI agents working together, like employees with distinct roles. Imagine calling a customer service center, where an AI agent identifies your need and routes you to a specialized AI agent based on your request. If it’s about a refund, you will be sent to the refunding agent, while if it is about a technical problem, you will be sent to the agent that is trained and fine-tuned as a technical support agent. The ‘swarm’ concept describes this coordinated capability, enabling agents to seamlessly hand off tasks and share information as required.
With recent advancements in voice technology—such as OpenAI’s ‘advanced voice mode’—these agents can now speak naturally, with real-time interruptions just like human interactions. OpenAI has released this voice functionality as an API, enabling businesses to integrate natural-sounding speech into any application, although initial high costs may limit its adoption.
2024 is also shaping up to be the year of the humanoid robot. Many companies, particularly in the U.S. and China, are competing to develop the first economically viable models. The advancements in humanoid robots over the past year are remarkable; their capabilities have evolved so rapidly that even industry insiders sometimes debate whether videos depict real robots or humans in suits (and we’ve seen both) and whether these robots are truly autonomous or teleoperated by humans.
These robots are expected to be priced similarly to cars and will likely be deployed first in factories for repetitive tasks. While Tesla has claimed that its Optimus robot is already operating in its car factories, this appears to be primarily a marketing move. However, we can expect pilots from several robot manufacturers by 2025.
#6. Everyone is Now a Programmer: How Is AI Transforming Traditional Job Roles and Skills?
The rise of AI agents and robots is just one part of the transformation; current employees are also becoming significantly more capable. In the short term, AI is expanding their skill sets across job roles. For anyone with over, say 20 years of domain experience, the productivity gains from generative AI is likely small. However, for employees with limited experience, AI enables performance on par with someone who has a few years of expertise—not just in one area, but simultaneously across a variety of functional roles.
For instance, AI has, in effect, turned everyone into a programmer. Consider an employee responsible for sorting, structuring, and submitting customer feedback to various systems—a task that typically requires an entire day each week. Traditionally, automating this would require IT department involvement, leading to months of evaluations, make-or-buy decisions, RFQ processes, budget approvals, and other tasks common in large corporations. Now, with a capable AI model, that employee can build the system themselves, using natural language. This shift raises key questions: How should this change be managed? Should employees be allowed to perform these tasks without IT oversight?
This shift isn’t limited to IT. Employees may no longer need Legal’s support to interpret a contract, assistance from business controllers to analyse financial data, or help from the People department to design a training program for upskilling. With AI, individuals across departments can independently tackle tasks that previously required specialized support.
AI adoption among employees will not be uniform. Some will quickly integrate AI tools into their workflow, achieving a boost in speed and performance, while others may resist adoption and continue at their current pace. High-performance individuals aren’t a new phenomenon—back in the 1960s, the ‘10x developer’ concept emerged, suggesting that some developers were up to ten times more productive than their peers, disproportionately impacting project success. The difference now is that AI has the potential to create ‘10x employees’ across every function.
Part III: Navigating the AI Safety and Risk Landscape
AI-empowered employees introduce new risks, as do employees without basic AI skills. Public and customer backlash is possible, and AI introduces a complex security and safety landscape at all levels of use. Risks include potential data mishandling, heightened cybersecurity threats, and the uncertainties that come with deploying new AI tools.
#7. Bring-Your-Own-AI: Are Your Employees' AI Habits Putting Your Company at Risk?
As employees discover they can boost productivity 10x with AI tools, the incentive to use the best tools—even without company approval—grows. The appeal of outperforming colleagues, coupled with unclear policies or prohibitions, may lead employees to keep their AI usage private. As a result, the primary beneficiaries of these tools are often the employees themselves, rather than the organization.
Unregulated AI usage can expose companies to data security risks, as employees may input sensitive information into consumer-grade tools with insufficient security. I expect that the risk of data leakage, in practice, is relatively low. A scenario could, for example, be an employee that enters proprietary source code into a free chatbot, that uses the input to train future models. In this case, if similar queries arise, the chatbot could potentially replicate aspects of that code, but that would likely be years later and subject to very specific conditions.
A more common risk is that employees inadvertently share data that is contractually protected or regulated under laws like GDPR. Organizations with greater AI maturity typically have clear guidelines on what types of data can be used with various AI solutions and under what conditions.
A straightforward countermeasure would be to ban all AI usage and strictly enforce this policy. However, this comes at a high cost. First, it would reduce productivity, both directly and in comparison to competitors. Second, it impacts employee retention. Employees who recognize that AI tools can boost their output 10x may feel stifled by restrictions, especially if competitors offer opportunities that embrace these tools. This creates a negative selection pressure, where the ‘10x’ employees may leave while the ‘1x’ employees remain.
#8. Expect the Best, Plan for the Worst: What Are the Real Risks of AI, and How Should You Prepare?
The risks associated with ‘1x’ employees who avoid AI extend beyond productivity. If they lack familiarity with AI, they become more vulnerable to next-generation cybersecurity threats. While it’s easy to imagine adversaries using AI to create sophisticated malware, AI is inherently dual-use—it can serve both defensive and offensive purposes. This introduces risk asymmetries, where AI’s potential for harm could exceed our ability to guard against it.
Most resources will go toward building safer IT systems and AI-based cybersecurity defences, far outstripping what criminals can spend on exploiting vulnerabilities. Yet, certain asymmetries persist; for example, it’s often easier to disrupt a system than to protect it, or to spread misinformation faster than it can be debunked. Consequently, well-protected servers in data centres may not be the primary risk—rather, social engineering attacks targeting employees unfamiliar with AI and its capabilities may pose the greater threat.
With AI tools and sufficient compute power, even a small team could disrupt an entire company—or play a pivotal role in swaying a close election. As models become more powerful, the potential to destabilize entities, even nation-states, through misinformation, manipulation, and cyberattacks grows, especially if they manage to do it in a way that also generates public support.
Beyond direct threats, companies must also consider reputational risks. AI initiatives can provoke backlash from employees and customers, particularly in areas where anti-AI sentiments are strong. Understanding these dynamics and preparing for potential resistance is essential as AI adoption continues.
Part IV: Embracing AI Personally
Navigating AI-related complexities requires a deep, hands-on understanding. The best way to gain this insight is through firsthand experience with the technology. Doing so not only enhances your knowledge but also sets a positive example for AI adoption within the company.
#9. Hands-On Leadership: How Should You Experience AI's Potential Firsthand?
Anyone can engage with AI through a chatbot—it’s as simple as interacting with a human and requires no special skills. However, the ability to ask the right questions is invaluable, and many senior decision-makers are already skilled in this, giving them a head start in leveraging AI effectively.
The secret to master AI’s capabilities is to test it across a wide range of tasks. It is not always intuitive what is easy and what is hard. AI can handle seemingly difficult things effortlessly, like diagnosing that persistent error message on your computer, or recommending the perfect wine for dinner, based on photos of the recipe and the available bottles. Yet, it struggles in other areas, such as analysing trends in polling data for an upcoming election, despite having access to all news and background information. Sometimes, though, these limitations can be circumvented by manually providing more context.
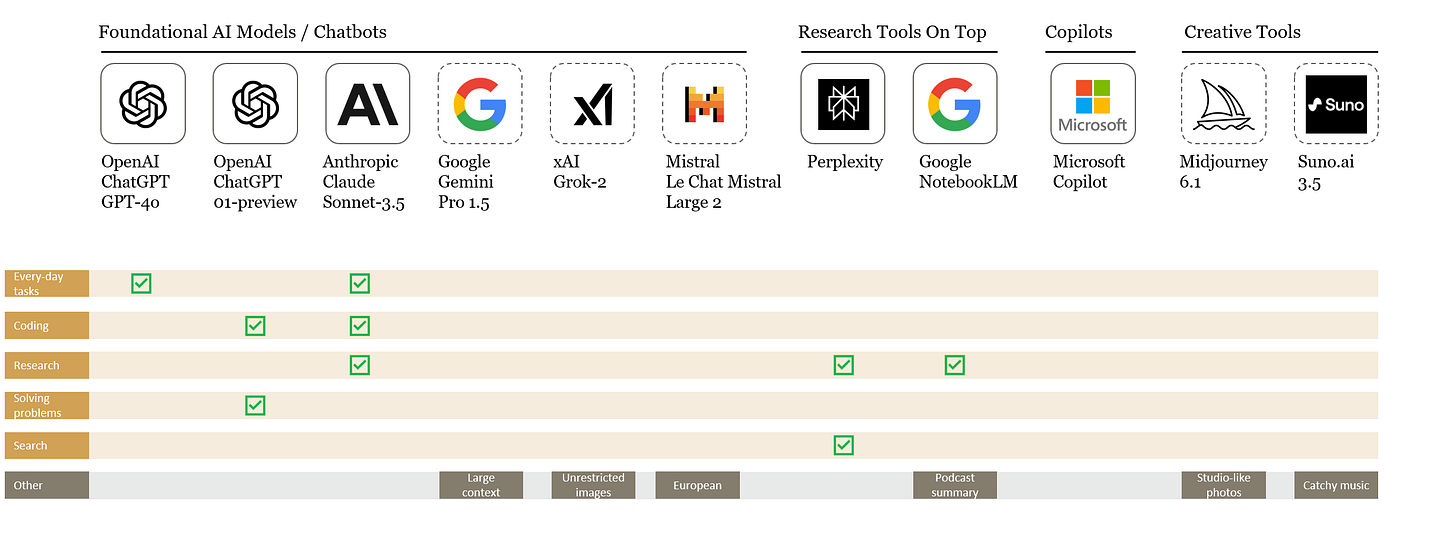
New tools are emerging daily, but having a core portfolio of familiar and reliable tools is invaluable. Many offer a free tier, though subscribing, if just for a month, can be worth it.
#10. Building an Edge: How Do You Use AI to Improve Decision-Making?
Generative AI can streamline tasks like drafting emails and summarizing reports, but its real potential lies in cognitively more difficult tasks, such as supporting in decision-making.
Current AI models are not yet capable of providing comprehensive, single-step recommendations for complex decisions. However, they can be invaluable for supporting in specific stages of the decision-making process.
A critical factor for success is recognizing that AI models need substantial context to address complex issues accurately. Providing sufficient context also helps minimize the risk of AI hallucinations. I often use AI to compile a ‘book’ of background information on a specific topic before addressing critical questions. This approach allows me to copy and paste relevant context into new chats to get the AI quickly up to speed. I can also build on that information over time, to deepen the AI’s understanding and improve the quality of responses.
For high-quality responses, models with advanced reasoning capabilities, often referred to as ‘system 2 thinking,’ are most effective. Currently, this means the OpenAI o1 model series, capable of solving problems at a graduate level. Although not all business problems require PhD-level expertise, this advanced capability unlocks valuable new use cases.
Statistics, for instance, can quickly become complex. Say you’re considering moving offices and want to be 95% certain that you understand the employees’ view on this—how many do you have to ask? Calculating the required sample size may be manageable if you’ve studied statistics, but it’s time-consuming, and most people would instead simply guess. With an advanced AI model, however, calculations like this can be completed in seconds.
Another valuable use case for AI in decision-making is scenario simulation. By testing various choices, you can gain insights into possible outcomes. For example, you might simulate customer reactions to a delayed product release, competitor responses, or shareholder impact. This approach provides countless opportunities to deepen your understanding of each scenario. The quality of these insights, though, depends on providing high-quality contextual information.
Additionally, LLMs are good at evaluating and ranking options, such as ranking a list of potential actions or evaluating risks. This offers an impartial assessment that can be challenging to achieve in decision meetings.
Finally, incorporating AI into decision-making allows management discussions to start from a more informed foundation. Discussions can quickly zoom in on the most critical and challenging topics, resulting in deeper and more productive conversations.
What is Next
We remain in the early stages of the AI era, yet the window for passive observation is quickly closing. Regardless of your organization’s level of ambition, a conscious and strategic approach to AI is essential.
In rapidly changing environments such as AI adoption, companies benefit from robust strategies designed to navigate diverse scenarios. Early steps should focus on ‘no-regret’ moves, such as building foundational AI knowledge and establishing usage frameworks. There are also low-hanging fruits in personal productivity, such as using AI tools for reading, writing, and research. Alongside foundational steps, consider investing in a few higher-risk initiatives that have manageable downsides.
With these basics in place, companies can progress up the AI maturity ladder and start integrating AI on a larger scale within digital transformation initiatives. While the stakes are higher, so too are the potential rewards. Now, more than ever, success hinges on speed, smart risk-taking, and dedicated, well-informed leadership.
A good place to start is to do my AI maturity assessment. The tool offers a personalized assessment, helping you gauge your AI maturity, identify your AI user type, and receive tailored recommendations for further learning based on your profile. For additional questions or support, you can reach out through PxS Advice.